Role of virtual simulation in surgical training
Introduction
Advantages in the use of virtual environments as training tools have been clearly shown both in military and civil aviation where simulation is now an essential tool for the training of pilots.
If on one hand it is easy to understand the reasons that pushed the development of effective simulators for the training in aviation (e.g., cost of aircrafts in addition to the cost of human life, among others), on the other hand it is not clear why surgery does not include standardized training curricula based on virtual environments. Aviation put lots of effort in developing and standardizing simulators for training since the first years of 1900 and has developed rules, guidelines and classifications for training curricula and training tools. In surgery, on the contrary, there is no consensus neither on the correct training curriculum nor on the capabilities that a proper training tool must have. However, the advantages related to the use of virtual environments in surgical training are manifold: they train the novice before he/she enters the operating room, reducing the number of errors which in turns reduces the intervention time and simplifies the follow up. In addition they can ensure a uniform training an objective and repeatable assessment of trainee skills. However the current limitations in their functionalities, the lack of relevant legislation and their improper use make training systems based on virtual reality underutilized tools.
The market proposes several training systems based on virtual environments for different surgical specialties, in particular for endoscopic surgery and robotic assisted surgery. Endoscopic surgery, in fact, requires the surgeon to look at the patient through a monitor; this greatly simplifies the reproduction of the intra operative environment. Robotic surgery allows further simplification, as the surgeon does not work directly on the patient; instead he controls two handles whose behavior is easy to reproduce with ad hoc devices (Figure 1).
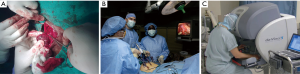
This simplification of the interaction between the virtual environment and the surgeon simplifies the analysis of the effectiveness of virtual environments in surgical training by reducing the number of variables to consider.
For this reason, this work focuses on robotic surgery training systems; however, the considerations related to virtual environments used for training in robotic assisted surgery can be easily extended to other surgical training systems based on virtual environments.
Robotic assisted surgery training systems
The market proposes five systems dedicated to the training of robotic assisted surgery. In alphabetical order they are: Actaeon, by BBZ srl; dV Trainer, by Mimic Technologies Inc.; RobotiX Mentor by 3D Systems USA Corp; ROSS, by Simulated Surgical Systems LLC; and SEP robot by SimSurgery AS. In addition to these systems it is possible to use the actual robot console to train in virtual environments thanks to the da Vinci Skills Simulator: an hardware module developed by the robot manufacturer Intuitive Surgical which can be attached to the robot console and that simulates the robotic tools and the environment.
These systems follow completely different approaches in the way they recreate the look and feel of the robotic console. A visual comparison of the systems is provided in Figure 2. Actaeon, ROSS and da Vinci Skills Simulator use robotics arms to reproduce the input devices, whereas dV Trainer uses a pair of cable driven input devices to get user input. RobotiX Mentor and SEP robot use magnetically tracked devices to get user’s hands pose and orientation.
Actaeon is the only training system which uses hardware specifically designed to reproduce the da Vinci control console whereas other systems integrates existing technologies.
During endoscope motion and clutching, da Vinci robots provides user with some guidance through forces and torques applied to master console handpieces. Forces are used to keep user’s hands at the same distance during endoscope motion, whereas torques are used to match user’s hands orientation with robotic tools orientation; da Vinci robot does not provide force feedback due to interaction between robotic tools and patient.
At the best of author’s knowledge, no systems but the da Vinci Skills Simulator provide torques during clutching and endoscope control. dV Trainer provides only forces during endoscope motion.
In addition to these differences in the hardware design, the systems have several differences from the point of view of the provided functionalities, their cost and their dimensions. A comparison of the key features of the systems can be found in Figure 3, the comparison does not take into account SEP robot, as no data about the system are currently available online.
It is worth noticing that all the systems but the da Vinci Skills Simulator, uses dedicated hardware. This means that the training center/hospital must reserve room for the training device. This is especially true for dV Trainer, RobotiX Mentor and ROSS, whose dimensions cannot be significantly be reduced by packing them.
Current technology limits
Virtual environments used in aviation greatly differ from the ones used in surgical training in terms of the provided realism.
A fully realistic training environment for surgical training should reproduce the interventional area with all the important structures, with anatomical variations, and realistic organ behavior. Virtual tissue should properly reproduce deformations during the interactions, but they should also integrate a biomechanical and functional model to predict damages (e.g., necrosis, blood loss...) and outcome of the simulated intervention. However the level of realism provided by the state of the art simulators is well below the aforementioned one.
As can be seen at https://vimeo.com/149546215, graphics realism of the reconstructed scene is good; however the behavior of soft organs is far away from being realistic. The deformation of soft tissue is exaggerated and thread penetrates soft tissue in several points.
There are two main limitations in the realistic simulation of interactive surgical environments. They are the modeling of biological tissue and the computation of the resulting equations. In fact, the simulation of organ deformations requires knowing the constitutive equation that relates the material stress and strain (1). Once the relation is known it is possible to compute tissue displacement as consequence of applied forces (2).
Biological tissues have extremely complex and rich constitutive equations, the complexity come from the structure and the composition of the tissue and depends, by many external factors, such as the presence of vessels, blood or fibers. For relatively simple materials it is possible to analyze their structure and to synthesize the correspondent constitutive equation. Currently, the only way to model the behavior of more complex tissue is to measure it and to identify the parameters of a suitable constitutive equation (3,4).
Measuring tissue behavior, however, is not trivial: the ideal condition to take the measures is in vivo but it raises obvious ethical issues and do not allow having complete knowledge of boundary conditions. Ex vivo and in vitro tests are also common, as they allow better modeling of boundary conditions, on the other hands the differences between the in vivo situation (the lack of blood flow above all) alter the response of the tissue.
Once the constitutive equations are known the next step is the computation of the stress/strain relation along the whole volume of the organ. This is commonly done by discretizing the volume and by approximating the value of the equation through numerical integration (5). Numerical integration is a computationally intensive task and is difficult to parallelize when complex behaviors need to be simulated. In addition, physics simulation requires additional collision detection and solution steps, which further increase the computation complexity. To ensure smooth and realistic simulations, however, the state of the whole environment should be updated at least every 16 ms (which results in a 60 Hz update frequency).
Several methods have been developed to speed up the simulation; some physics engine (software libraries specifically developed to perform physics simulation) can also take advantage of the computational power provided by graphics cards (https://www.youtube.com/watch?v=1o0Nuq71gI4). However state of the art techniques associated with common hardware still do not provide realistic simulation of surgical gestures in complex environments.
Approaches to training
The lack of requirements and guidelines about robotic surgery training gives extreme freedom to trainer manufacturers and to hospital and training centers about the implementation of training curricula. For this reason there are evident differences in the approach followed by the training systems on the market.
All the systems provide basic skills training tasks, to teach the trainee visuo-motor coordination, endowrist manipulation and how to effectively control the robot by properly using footswitches and finger clutch. dV Trainer and RobotiX Mentor are the only feature procedural training, i.e., training to surgical procedures or part of them.
Actaeon stresses the realism of the physical simulation, as a mean to provide effective training. The rendering of the scenes it proposes is not photorealistic and the environments do not mimic human anatomy. However the physics of the environment and the interactions between objects and tool are realistic: objects do not compenetrate. This realism translates in more accurate simulation for example, and to the best of author’s knowledge, Actaeon is the only training system that simulates thread snapping in knot tying exercises. An example of a training task of Actaeon is provided at https://www.youtube.com/watch?v=xiQYL-P1PVg.
dV Trainer, on the other hand, proposes simulations with realistic graphics rendering and anatomical environments. dV Trainer features some procedural training tasks in which organs are modeled with linear stress/strain relation (i.e., tissue deforms unrealistically when stretched or punctured). It includes the Maestro AR module, which reproduces the recording of real interventions. During the playback the user is asked to identify structures or corrects action through multiple-choice questions (one example of the tasks proposed by this module is presented at https://www.youtube.com/watch?v=xiQYL-P1PVg). Movie at https://www.youtube.com/watch?v=jgsBeV0Pl1o shows an anastomosis task simulated by dV Trainer. dV Trainer integrates a team-training module which allows the first operator and the assistant to cooperate for the completion of some tasks.
RobotiX Mentor further increases the graphics realism of the simulated scenes, with photorealistic images and complex anatomy reproductions. On the other hand it provides less realistic physics modeling with respect to Actaeon and dV Trainer, this results, for example, in objects that easily compenetrate. Robotix Mentor, however, includes the most complete set of procedural training tasks (one of which is shown in https://vimeo.com/149546215). Like dV Trainer, also RobotiX Mentor features a team-training module.
Although ROSS manufacturer presented the new model of the system, no information is available about the simulation software, thus this paper refers to the old model. ROSS graphics realism is limited, as it is limited the realism of interactions and robot kinematics and dynamics. ROSS integrates a unique training tool that playbacks recorded movies of surgical task and move trainee’s hands according to the position of surgeon’s hand. It is worth noticing that only position of hands and not their orientation is guided during the playback. One task provided by ROSS is proposed in https://www.youtube.com/watch?v=Pv83P9EqC_0.
The software that runs on the da Vinci Skills Simulator integrates many training tasks from dV Trainer and few from RobotiX Mentor, but it misses the procedural tasks. For this reason the experience and the training curriculum provided by the da Vinci Skills Simulator is very similar to the one provided by dV Trainer.
Since there is no consensus neither on the required training tasks nor on the required level of realism, the robot manufacturer is supporting a project whose goal is the definition of a basic curriculum for robotically assisted surgery training, the main characteristic of the curriculum is that it is independent from the simulation modality, this means that the tasks included in the curriculum are valid both in their real and virtual versions and that their effectiveness does not depend on the virtual simulator used (both dV Trainer and RobotiX Mentor are involved in the project) (6).
The complete results of the study have not been presented yet, however the tasks identified for being included in the curriculum involves only basic skills, unrelated from any specific surgical procedure or anatomy (Figure 4 and https://www.youtube.com/watch?v=GVxeM5Biry0). In addition, at the best of author knowledge, the study does not clearly describe the minimum level of realism provided by the software or the properties of the soft tissues involved in the simulation (https://vimeo.com/106466489).
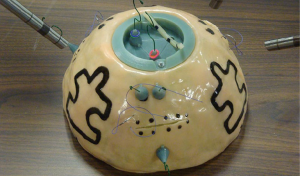
Conclusions
Although virtual environment are commonly considered useful instruments for the training in robotic assisted surgery and, in general, for minimally invasive surgery their use has been regulated neither by national governments nor by surgeons’ societies. This leaves training system manufacturers without guidelines and clear requirements and has led to the development of training systems that greatly differ in terms of hardware functionalities and virtual environment characteristics.
One important aspect to consider, however, is that it is currently very hard, if not impossible, to faithfully reproduce the complexity of the human anatomy involved in a surgery. This makes even more important the definition of the minimum requirements in terms of realism that training systems have to provide to be effective.
There are few ongoing projects that want to identify the skills required to safely carry on a robotically assisted intervention, to define the tasks that should be used to get these skills and the metrics used to evaluate the proficiency of the trainee. The fact that these projects focus on relatively simple simulated tasks may be due both to the difficulties related to the identification and evaluation of surgical skills or to the limitation in terms of trained skills of current simulations.
The future goal, for all the training system manufacturers, is to obtain very realistic simulations, to allow surgeons training in complex anatomical environments with realistic tissue and organ behavior. This way it would be possible to completely replace the experience on real patients and thus to shorten the training process, by proposing very specific cases with high educational value which cover the principal anatomical variations and pathologies.
Acknowledgements
None.
Footnote
Conflicts of Interest: D Zerbato is partner of BBZ srl, a company which produces and sells training systems for minimally invasive surgery. And have no conflicts of interest to declare.
References
- Fung YC. Biomechanics: mechanical properties of living tissues. New York: Springer-Verlag, 1993.
- Cook RD, Malkus DS, Plesha ME, et al. Concepts and Applications of Finite Element Analysis. Hoboken: John Wiley & Sons, 2001.
- Chen H, Zhao X, Lu X, et al. Non-linear micromechanics of soft tissues. Int J Non Linear Mech 2013;58:79-85. [Crossref] [PubMed]
- Pawlikowski M, Klasztorny M, Skalski K. Studies on constitutive equation that models bone tissue. Acta Bioeng Biomech 2008;10:39-47. [PubMed]
- Cioacal T, Caramizaru H. On the impact of explicit or semiimplicit integration methods over the stability of real-time numerical simulations. ROMAI J 2013;2:75-87.
- Fundamentals of robotic surgery. Available online: http://frsurgery.org/, accessed 3 November 2016.
Cite this article as: Zerbato D, Dall’Alba D. Role of virtual simulation in surgical training. J Vis Surg 2017;3:23.